Ethical AI and Bias Mitigation in Hiring
We aim to understand how hiring managers and hiring agencies, who license off-the shelf models and have no influence or control on how the model has been trained, select and use source data for collecting, screening, and selecting candidates for interviews. By examining their methods, we hope to identify potential issues and develop improvements to eliminate any biases in their system.”
Problem Statement
“We aim to understand how hiring managers and hiring agencies, who license off-the shelf models and have no influence or control on how the model has been trained, select and use source data for collecting, screening, and selecting candidates for interviews. By examining their methods, we hope to identify potential issues and develop improvements to eliminate any biases in their system.”
Problem Background
During the early 2010s, pioneering companies began experimenting with AI-driven recruitment tools to streamline various aspects of the hiring process, such as resume screening and candidate sourcing. From the mid to late 2010s, the adoption of AI in hiring accelerated significantly. As AI technologies matured and became more accessible, companies across various industries started leveraging AI-driven solutions to enhance their talent acquisition processes. In the 2020s, AI has become increasingly integrated into mainstream hiring practices. Companies of all sizes and industries are now leveraging AI-driven solutions to address common recruitment challenges, such as high volumes of applicants, time-intensive screening processes, and biased decision-making.
The COVID-19 pandemic further accelerated the adoption of AI in hiring, as remote work and virtual recruitment became the norm, leading companies to seek efficient and scalable solutions to manage their recruitment needs.
Today, AI is a ubiquitous presence in the hiring process, with an increasing number of companies relying on AI-driven tools and platforms to augment their recruitment efforts.
According to a 2021 survey by Gartner, 43% of organizations reported using AI in their hiring process, representing a significant increase from previous years. Additionally, 69% of organizations planned to increase their investment in AI for talent acquisition over the next few years.
While AI can significantly enhance productivity and efficiency in companies, it is crucial to recognize that AI is not a panacea. Companies using off the shelf AI models must acknowledge the existing biases in AI data and the need for continuous training and improvement. It is their responsibility to ensure ethical and responsible use of AI by partnering only with well-trained data agencies who focus on mitigating bias and ensuring fairness of hiring outcomes.
Studies by The Greenhouse Survey on AI in Hiring have shown that 80% of companies use AI in their hiring process, but about half of them surveyed do not actively monitor their AI tools for biases. The World Economic Forum reports that only 25% of organizations have policies in place to ensure the ethical use of AI, indicating a widespread lack of governance in this area.The failure to effectively mitigate bias in off-the-shelf AI hiring models can have far-reaching and severe consequences such as:
Discrimination Against Certain Demographics:
- Gender Bias: Amazon scrapped its AI recruiting tool after it was found to favor male candidates over female candidates. The tool was trained on resumes submitted over a 10-year period, which predominantly came from men, leading the AI to favor male-dominated language and experience .
- Racial Bias: A study by The National Bureau of Economic Research found that job applicants with "Black-sounding" names were less likely to receive callbacks compared to those with "White-sounding" names, even with identical resumes . If AI systems trained on biased data perpetuate these patterns, racial minorities are unfairly disadvantaged.
Loss of Opportunities:
- Resume Filtering: AI tools that are biased against certain keywords or experiences might filter out highly qualified candidates. For instance, AI systems trained on past hiring data may inadvertently disfavor candidates from non-traditional educational backgrounds or career paths, limiting diversity and innovation.
Workforce Homogeneity:
- Lack of Diversity: A biased hiring process can result in a less diverse workforce, limiting the variety of perspectives, experiences, and ideas within the company.
Employee Morale and Retention Issues:
- Lower Morale: Existing employees may become disillusioned if they perceive their company as unfair, leading to decreased job satisfaction and engagement.
Social and Ethical Implications:
- Contribution to Systemic Bias: Continued use of biased AI models in hiring can perpetuate systemic biases in the job market, contributing to broader societal inequality.
Internal Culture and Conflict:
- Internal Conflict: Perceived unfairness in hiring practices can lead to internal conflicts and divisions within the workforce, affecting teamwork and collaboration.
Specific Examples and Statistics
- Amazon’s AI Tool: Amazon abandoned an AI recruiting tool because it penalized resumes that included the word "women's," as in "women's chess club captain" .
- HireVue’s Facial Analysis: HireVue, a company providing AI-driven hiring assessments, faced scrutiny and criticism over potential biases in its facial analysis technology. The company eventually ceased using facial analysis due to concerns about fairness and bias .
- Risk of Automation Bias: Research from the AI Now Institute highlighted that reliance on automated systems could lead to automation bias, where humans overly trust AI recommendations even when they are flawed, exacerbating biased outcomes.
User Pain Points & Research Insights
After searching 6 sites ( sources listed below), From over 2000 participants of surveys and studies done — HR professionals and executives spanning various industry sectors small to large organizations, I concluded on 3 main key areas highlighting the problem of bias in AI-based hiring processes for companies using off-the-shelf models.
1. Although almost 80% of companies use AI in their hiring process, about half of them surveyed do not actively monitor their AI tools for biases.
2. 60% of them had positive feedback and appreciation for the difference AI is making with regard to improving on the hiring process however they express concerns about the lack of transparency with the tools and no control over training data, hence potential biases.
3. 67% of the companies surveyed are now making efforts to enhance training data against biases, however 80% still stress on the need for human oversight to detect and prevent biases, with 69% in support of the creation of regulatory standard frameworks emphasizing that unchecked AI tools can lead to unfair hiring practices.
Supporting Data
Should be noted that percentages given is the average of all results of studies and surveys cited.
- Screening Applications: 72% of companies use AI for initial pre-screening, parsing, ranking and shortlisting of candidates.
- AI Systems/Tools Used: Game based assessments, ATS systems and video interviews platforms, HireVue, Pymetrics, IBM Watson, SAP SuccessFactors, LinkedIn Recruiter.
- Last AI Tool Usage: 53% had positive feedback on efficiency but mixed feelings on fairness.
- Likes/Dislikes: 62.5% appreciated automation and reduced time-to-hire, while 32.5% raised concerns over lack of transparency and potential biases.
- Model Training/Retraining: All studies stated none-to-minimal control over training data, and emphasized the need for diverse training data and regularly auditing AI outputs with balanced data sets.
- Efforts to Improve AI Systems: 67% are taking steps to enhance AI fairness through feedback incorporation, improved data diversity, bias audits and model updates.
- Human Oversight: 79% believe human oversight is crucial in identifying and mitigating biases in AI hiring decisions.
- Regulatory Standards: 69% support the creation of regulatory standards to ensure ethical AI practices in hiring.
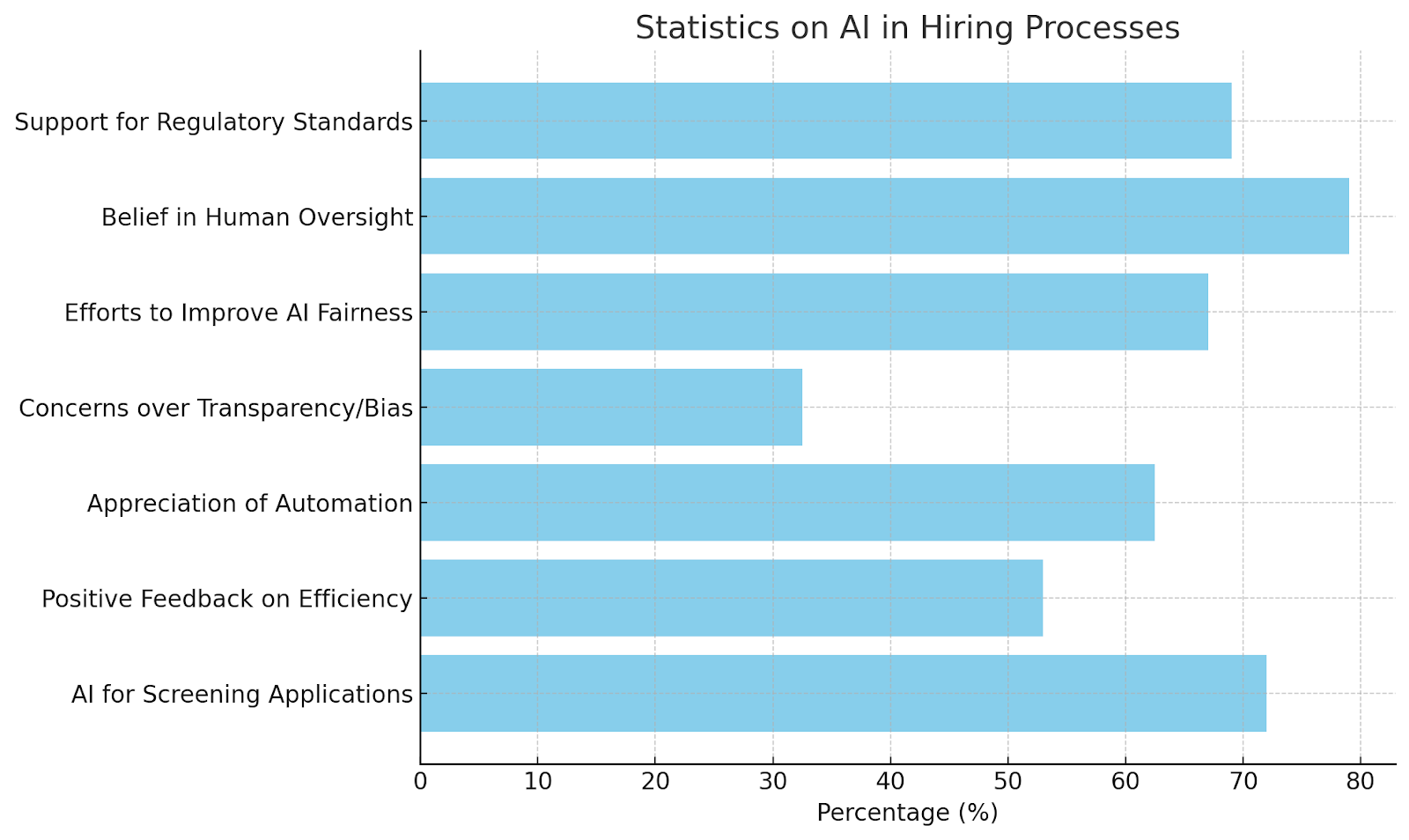

2019 (MIT Sloan): 800 participants
- This research examines the fairness of AI systems used in hiring practices.
2020 (Deloitte): 1,200 participants
- This survey focuses on the adoption and impact of AI technologies in human resources (HR) functions, including recruitment and talent management.
2021 (HBR): Estimated 100 participants
- This reveiw report focuses on the intersection of AI and bias in the hiring process.
2022 (Brookings): Estimated 200 participants
- This research report focuses on the challenges associated with mitigating bias in algorithmic hiring processes.
Feedback
From the research findings, we can conclude that mitigating bias in hiring with AI is imperative and many companies still have a lot of work putting in place effective strategies to fix this problem.
Landing on the Solution
Based on our target’s pain points, I would like to propose actionable steps to work on the problem by addressing the following key areas:
- Collaborate with AI Vendors for Customization: Engage AI vendors in discussions about bias and fairness, and negotiate for customizations or adjustments that align with the company’s ethical standards and diversity goals.
- Enhance Transparency and Explainability: Only partner with AI vendors that utilize AI models that provide clear, understandable explanations for their decisions, and share these explanations with candidates and hiring managers.
- Integrate Human Oversight and Review: Create protocols where human recruiters review and validate off the shelf AI-driven hiring processes and decisions.
- Implement Candidate Feedback Mechanisms: Develop and maintain feedback systems where candidates can report their experiences, and share this feedback with your AI Vendors so they can continually improve AI hiring and human decision-making processes.
Explanation of Solution
From the research I carried out, it shows that 67% of companies are beginning to implement some of the above stated solutions to mitigate bias in AI hiring. However consistency and effectiveness is key. The goal is to reduce the gap as much as possible for the coming years. More intentionality is needed if we want to see a significant change with mitigating bias in hiring. A few more forward thinking suggestions that can bring about more effectiveness to solving this problem are as follows:
Algorithmic Fairness Certification: Companies using off the shelf AI models should advocate for the establishing of independent certification bodies or standards organizations tasked with evaluating the fairness and reliability of AI models used in hiring processes. By patronizing agencies with algorithmic fairness certifications, companies can demonstrate their commitment to ethical AI practices and build trust with candidates and stakeholders.
Blockchain for Data Traceability: AI agencies should consider utilizing blockchain technology to create an immutable record of data transactions and model updates throughout the AI lifecycle. By providing transparency and traceability, blockchain can help ensure the integrity and fairness of AI-driven hiring decisions while enhancing accountability and auditability.
Personalized Fairness Constraints: Hiring professionals and executives should champion the development of personalized fairness constraints that account for individual preferences and circumstances, allowing candidates to specify fairness criteria relevant to their unique backgrounds and experiences. This approach empowers candidates to advocate for fairness in the hiring process based on their specific needs and concerns.
Future Steps
By the end of this sprint, It became clear to me that though I got clarity about my problem statement, implementing a solution was not going to be feasible from my end. I have understood that mitigating Bias in hiring while incorporating AI will take more effort and will need a lot of time. I am glad companies are already working on solutions to solve the problem and that more awareness is being raised. However, I will not be moving forward with working on this because I don’t currently have the capacity to do so. If my suggestions for achieving more effectiveness with regard to the solution of this problem are found valuable sometime in the future, I will be glad to participate in whatever way that is accorded me.
References
- The Greenhouse Survey on AI in Hiring: https://www.fastcompany.com/90989496/ai-bias-hiring-managers
- Brookings Report on Algorithmic Hiring: https://www.brookings.edu/articles/challenges-for-mitigating-bias-in-algorithmic-hiring/
- McKinsey Report on Mitigating AI Bias: https://www.fastcompany.com/90989496/ai-bias-hiring-managers
- AI and Bias in Hiring Report by Harvard Business Review (HBR): https://hbr.org/
- Research on AI Fairness in Hiring by the MIT Sloan Management Review: https://sloanreview.mit.edu/
- Report on Algorithmic Bias in Hiring by the Brookings Institution: https://www.brookings.edu/
- Survey on AI in HR by Deloitte: https://www2.deloitte.com/
Learnings
Product Manager Learnings:
Nora Galabe
This sprint was my first attempt at acting in the role of a Product Manager and I must say it’s been an eye opener. For starters, it entails more than I had imagined.
- It is key to understand the ins and outs of a problem in order to provide the right solution. You must remain focused on the problem at all times if you want to build the right product.
- Also, research skills are paramount to understanding what your target group really wants. It's not just about asking questions, but asking the right questions using the right methods based on the characteristics of your target group. Asking questions is not enough, you have to go to the next level by probing as well in order to really understand your target group’s fundamental desires with regards to the problem you are trying to solve. I believe understanding human psychology will give you an edge here. It’s not just what they say, but how they act.
- This sprint has taught me discipline and time management while attempting to achieve a goal in a short timeframe. I am glad I took on the experience and I hope that I can leverage the skills and lessons I have learned throughout this process in other future projects.
- Lastly, I want to thank my mentor, Lisa Nguyen for the very thoughtful and detailed feedback she gave me throughout the sprint. Two very valuable lessons I learned from her feedback are:
- Stay focused on your problem statement and make sure that you are aligned to it every step of the way.
- Details are important in research. Do not leave any information out that could cause ambiguity for those trying to understand what you are trying to achieve.
I now understand that becoming an effective and successful PM requires a lot of focus and attention to detail.
Designer Learnings:
Designer Learnings:
Jo Sturdivant
- Adapting to an Established Team: Joining the team in week 6 of 8 was challenging, as I had to quickly adapt to existing workflows, dynamics, and goals. This mirrors real-world situations where you often integrate into teams mid-project, and flexibility is essential.
- Work-Blocking for Efficiency: With only two weeks to complete the project, I learned the importance of a structured work-blocking system. This approach allowed me to manage my time effectively and meet deadlines under pressure.
- Making Data-Driven Design Decisions: Unlike my past projects, I had to rely on research conducted by others. This was a valuable experience in using pre-existing data to guide design decisions, helping me focus on the core insights without starting from scratch.
Developer Learnings:
Developer Learnings:
Vanady Beard
&
As the back-end developer, I learned how important it is to create efficient and reliable systems that support the entire application. This experience also taught me the importance of optimising the database and ensuring the backend is scalable and easy to maintain.
Developer Learnings:
Stephen Asiedu
&
As a back-end developer, I've come to understand the importance of being familiar with various database systems and modules. This knowledge enables me to build diverse applications and maintain versatility in my work. I've also learned that the responsibility for making the right choices rests on my shoulders, guided by my best judgement.
Developer Learnings:
&
Developer Learnings:
Maurquise Williams
&
- Process of Creating an MVP: Developing a Minimum Viable Product (MVP) taught me how to focus on delivering core functionalities balancing between essential features and avoiding scope creep.
- Collaboration in a Real-World Tech Setting: This experience taught me how to collaborate efficiently in a fast-paced tech environment, keeping the team aligned and productive, even while working remotely across time zones.
- Sharpening Critical Thinking and Problem-Solving Skills: This experience honed my ability to think critically and solve problems efficiently. By tackling challenges and finding quick solutions, I sharpened my decision-making and troubleshooting skills in a dynamic, real-world setting.
Developer Learnings:
Jeremiah Williams
&
All in all this experience was very awesome I learned that in coding with others being transparent is key
Developers Learnings:
Justin Farley
&
I learned how important communication is when working with a team. Communication provides understanding, advice, ideas, and much more. While working with the product team, I’ve found that communication keeps everything flowing smoothly. Working with a team also showed me that every member brings something different to the table and we all have to work together in order to align and meet our end goal.